- Viewpoints
- Posts
- AI in Gaming: Hype Or Reality?
AI in Gaming: Hype Or Reality?
A look at the past, current and future of AI in gaming
This is the first in a series of posts written by FOV Ventures Entrepreneur-In-Residence Tiago Correia that take a deep dive into various topics we’ve been exploring at FOV Ventures, taking a look at their history and some of the new startups building within the area. Tiago was previously an investor in Anything World and operator at Move.ai which are both referenced in the below article.
It’s 1997. The 12th of May. Doesn’t matter where you were. Because wherever you were, if you had access to a TV, or a radio or a newspaper, it would be featuring these words: “Computer Beats Chess Grandmaster”. It was the day after IBM’s famed DeepBlue had finally won against chess champion Garry Kasparov. The machine’s revenge, as some would have called it, after it had failed to win with a 4-2 loss just a year prior. Images of Kasparov’s furrowed brows, in intense concentration, were shown all across the world, in a heralding that even our brightest and best did not stand a chance. If it had been today, Deep Blue would have gotten a fashion brand endorsement on its TikTok page.

Kasparov vs AI
Fast forward a few months to October and the battlefront is a different one. You’re at your computer, playing this game called “Age of Empires”, that somehow the maker of Windows decided to put out. And you quickly find out that if there’s a damaged enemy building, even if it’s in a zone that you completely control, the ‘computer’ will keep sending villagers to repair it. No matter what! So you exploit that dumb weakness to the maximum. As well as others that you find along the way. This stark difference of experiences of ‘AI’ in games serves to highlight that we, as humans and as the arbiters of any other intelligence than our own, have been pitted against the machine for as long as we can fathom that concept. And that that relationship is a fluid one, where sometimes the Turing test is passed. And sometimes the uncanny valley is uncrossable.
It’s 2023. By the time we’re writing this and if there’s one topic on everyone’s minds it’s ‘AI’. Even the memes about such saturation are by now unbearable. This is also, certainly, not the first piece a VC writes about ‘AI’ in Gaming as well. There. Have. Been. Many. And the reason for that excitement is simply because the pace at which the underlying technologies and infrastructure supporting ‘AI’ is advancing, have allowed a significant leap. And certainly one of those leaps which will remain in the annals of history.

Source: Chris Ume
We’ve seen deep fake Tom Cruise turn into a hyped start-up, called Metaphysic AI, that’s now servicing Hollywood. We’ve seen ChatGPT pass the bar exam. And in gaming, VC excitement is outmeasured only by the valuations some companies are being invested at. But it is inconspicuously off-put by the caution developers often demonstrate for the same companies and technologies. This is usually chalked up by some as mere ‘conservatism’ - not wanting to change the way things are done - many times mixed with a sense of ‘self-preservation’ - switching to something you’re no longer the expert in is scary. But we’d like to give a bit more credit here to game developers. After all, they have been using some shape of the wide label that is ‘AI’ for quite a long time.
So where does the tension between dreamy excitement and a good ol’measure of realism come into this debate? For that we need to look at the three main areas where we’re seeing deep learning models being put to use in the gaming sphere:
1. In-Game Intelligence
In-game intelligence is the oldest area in the field, with academics going so far as illustrating uses of advanced game intelligence as far back as the 50s. What we mean by in-game intelligence is a broad bucket, but can mainly be categorised as the actions or reactions the game takes, via its version of players, characters, objects, environments, etc. Historically, most games tended to be multiplayer affairs, often placing one player against another, or one team against another. Typically, although not exclusively, with one side against another. So when video games appeared, the natural proclivity of these were to mirror the approach taken. While some allowed for that PvP setting, particularly in the domain of the arcades, almost all of them conceived of a world where only one P was available. Thus, the emergence of an always-ready intelligence, that could face up to the player upon request, was a necessity. It wasn’t just luck, like the random shuffle of a Solitaire card deck. The machine could employ differing approaches in scaling difficulty, like increasing/decreasing health, damage and DPS (damage-per-second), or even more complex methods like storing variables that represented different techniques, tactics or game-plays which could be called upon at random times or even because of certain player-induced triggers.

The Nemesis System from “Shadow of Mordor” Source: Warner Bros. Interactive Entertainment.
But solo-playing was still, very much, a social experience, with leaderboards and highscores turning into the de-facto currency of skill in a particular game. What the machine would throw the player at any given time became the stuff of legend, with memes like ‘Nuclear Ghandi’ as a classic result of an unplanned integer overflow, all the way to beloved experiences like the memory of orcs in “Shadow of Mordor”. In fact, overthrowing the designs of the developers quickly became an industry in its own right, with early video-game magazines all the way up to websites like IGN offering wikis, walkthroughs and strategy guides to overcome certain challenges.
Behaviour
When it came to designing these challenges, via the use of enemies or NPCs, behavioural decision-trees had been the most common route, with decisions being made at each node, depending on what was happening at any given time. The classic example is Halo, which even took into account the prioritisation of decisions in certain contexts - e.g. health is low so, instead of charging against the player guns-blazing, I’ll take cover. Another common approach is the one perfected in “F.E.A.R.” with goal-oriented action-planning (GOAP). This relies on one or more goals being assigned to a particular enemy. Depending on their priority, to achieve these goals, the machine will routinely search for what is the best permutation of actions, available to that character at any given time - this is the plan. But plans change. Plans have to change. Because the environment and the game state is also constantly being changed - e.g. a door is now closed - and so the machine needs to re-evaluate, at every step of that plan, from right after the plan is conceived to after the actions have been executed to check if they’ve been properly executed. These are multiple calculation requests, happening constantly, per character that has this programming in the current game state. This is made possible due to the existence of large in-game databases, locally stored alongside the executable files of the games, which are quickly called upon for its values, or even by reserving some memory for said compute.

Beating Humans at Capture the Flag Source: DeepMind
With modern deep learning approaches, the scale of data points potentially analysable and decision nodes employable is unvimaginable. In the absence of clear parameters, the ‘AI’ challenger could indeed take surprisingly smart decisions like concentrating all the enemy characters defending the final checkpoint of a game map. Or even worse, with machine learning, the behaviour of a player could be learned upon, to predict it, just as a next token is predicted in inferences, and successfully counter it. Hell, if the game is online, the whole collective behaviour of players could be drawn upon to ante up the challenge - instead of having them all concentrating in the finish line, they could now be waiting for the player with a trap! This poses a core game design problem: is this how the developers intended the game to behave? It’s in this field that play testing companies have existed historically and now, startups like Copenhagen-based modl.ai are innovating. Allowing reinforced learning to predict how players could behave based on certain programmed rewards and actions and let those ‘AI’ agents roam free in the world to see what happens, testing their design, before coming in with their invisible hand to tweak and iterate it.

Source: Cinnamon AI
Part of this debate is the reason why another form of design, hierarchical task network planning (HTN), was introduced into game design. Because it is actually more ‘design’. It allows more nuance from the developers by structuring dependencies between actions as well as how they can be grouped according to cost, in tasks, chained up to solve a higher-level goal. For example, a developer might not want the machine to overwhelm a player with all its enemy forces charging forward gung-ho, because they might want to spend one turn healing up and one further turn after that setting up mines, before finally spending a final turn actually engaging with the player. It’s precisely this balance between a machine’s free agency to make decisions vs. the input of a game designer that is raging in the debate of using LLMs for NPC dialogue interactions. When one is planning enemy - read hostile - behaviour in characters, the number of actions are always quite finite: its impetus will mostly be to fight, maybe flee, and as described previously, the number of actions associated with them are quite finite, maybe fighting will include a few types of attacks, and fleeing will just be running, maybe healing or hiding. But when the behaviour is not meant to be hostile, well then, the ‘AI’ has a whole world of options. Machine interaction with the environment is certainly fine if a developer is programming very specific rewards and goals. There is that instantly classic Stanford and Google study that recreated a village with 25 ‘AI’ agents populated in it and they all decided to throw an election, in a lovely show of virtual democracy. But most of the time, simulated ecosystems have stuck with some stable behaviours like “Red Dead Redemption 2”’s NPCs which have jobs to go to, houses to sleep in, and favourite saloons to drink in. Players are meant to disrupt that with their actions, but mostly the same set of tasks are generally chained up after any player-induced disruption.
Dialogue
The same finite number of options has usually been replicated when it comes to dialogue. Role-playing-games tend to be known for having a lot of dialogue, some which might even impact the gameplay - the classic example being evil vs good dialogue options. So when you introduce modern-day LLM layers such as ChatGPT, as InWorld is doing, to provide the NPC chat-backbone of a game, there’s a potential for all hell breaking loose. First off, in a truly generative approach, it’s impossible to predict what might come out of the mouth of that NPC next: forget if it’s relevant, can it be completely offensive? But even if there are morality guardrails, and if the training dataset of focus for context is mostly the game’s lore, what happens when we transform a previously discrete player input to an open-ended text box where players can say anything? How can NPCs understand the madness that players will tell them into a coherent interaction? This is not just a natural language (NLP) problem but a design one. Developers can’t simulate millions of potential dialogue options for wildcards, like they do with enemy actions on a map. This is why startups like UK-based Meaning Machine are hoping to work closer with developers, going hand-in-hand with the game design function, so that there’s a balance between player choice and machine reaction. Because the truly exciting thing to look out for is when we can expect NPCs to have a level of contextual awareness that they can start understanding open-ended player commands, can interpret them without confusion, and can behave in a way that the player understands, even if that behaviour ends up being actions instead of dialogue: imagine being able to play any of the “Total War” games where you can command your troops with your voice, just like a real general would? Sadly, the access to that level of foundational model is still cloud-stored which creates the classic delay problem for interactions. That might not be a problem for the real-time-strategy genre, but for RPGs, open-world and first-person-shooters (FPS), you really don’t want that lag to break your immersion, or else, get you shot. Locally-stored, maybe even fractionally-sliced LLMs will need to be created to be able to provide these features at a level that does not impact player satisfaction, and we’re yet to see that in the market yet.

Source: Replica Studios
While we’ve seen the conclusion of the WGA strike in Hollywood, we’re still to see how the SAG-AFTRA one concludes. And we already know the next target for the WGA - the games industry. So we are sure to be seeing the same concerns around ‘AI’ from the two guilds, when they collectively make their way to the games industry. And if we’re to believe that they will come emboldened from a hard-fought and hard-won victory from Hollywood, we can only assume that they will bring similar demands around script-writing, vocal synthesis, likeness-based avatar creation, etc.. But in a medium which is interactive by default and by comparison to Film and TV, it’s going to be interesting to see how those demands shake out, adding a layer of uncertainty about these solutions.
2. Game Development
One of the most exciting areas where the field is advancing extremely rapidly is actually on the development and design side. While we mostly spoke about design when talking about programming action and dialogue for the game to interact with the player, in this section we’re talking about the pieces that come before that. We’re talking about asset creation, from visual to audio, to rigging and animation, even to gaming mechanics. The entire workflow pipeline has been made significantly quicker and easier than previously manual and quite labour-intensive options. Not surprisingly, this is the section where the excitement of VCs and developers is more aligned. Again, it’s not like the use of ‘AI’ in this field was created in a vacuum. There have been instances of world-creation which used procedurally-generated content (PCG), for whatever reasons: every time you start a game in “SimCity”, “Dwarf Fortress” or “Minecraft” the map you had to build on was different, to encourage replayability; “No Man’s Sky” famously has an infinite universe, filled with countless planet permutations; and the old-school “The Elder Scrolls: Daggerfall”, was able to have a map the size of the UK, intelligently saving file-size by having the game generate hundreds of ‘filler’ villages in-between actual points-of-interest. These approaches were typically run via the use of some basic building-blocks being randomly assigned locations with certain quotas and rules, to generate maps or locations. But developers still had to conceptualise the assets and design them. Until now.
Asset generation
Because of the advances in generative neural networks and diffusion models, the process of conceptualising art ideas from nothing has been made much quicker. Art directors can use existing foundational tools like Midjourney, Stable Diffusion, etc. sometimes often for free, to help them come up with ideas. Ideas that they don’t have to manually mock up, but rather come illustrated with acceptable levels of output-quality. Now, these are just 2D mock-ups and for a lot of art directors, mock-ups for the purpose of conceptualization is what they are looking for. But it’s important to note that, despite the percentage being relatively small (and decreasing), for the 36% of the games developer market which develop fully 2D-games, this solution is really all they need, so naturally companies like Artbreeder and Scenario (an FOV investment) have popped in to service that section of the market, using generative adversarial networks (GANs) to deliver higher-than-acceptable levels of quality in their output, allowing developers to create anything from 2D potion icons to to 2D familial cats.

Source: Scenario
Some art directors, though, might be right in saying that a consumer’s sensibility is more attuned than we might think and that a game made solely of randomly generated AI assets might look at best, a bit kooky, at worst a hodge-podge of weird art styles splashed together. But if you have a strong enough reference data-set, developers can run style transfers to make sure that assets follow the distinctive style that art directors want. That still leaves a significant portion of the market out in the cold: those who are building 3D games. This is where the beauty of neural radiance fields (NeRFs) and similar approaches are coming in. Companies like Luma.ai have been allowing for the capture of real-world objects into 3D, with just a simple video capture on a phone, and FOV portfolio company M-XR, pushing the envelope in capturing real-life materials into 3D textures. The natural limitation here is that not everything you want to put in a game exists in the real-world though. Which is why diffusion models are being used to generate different 2D angles of the same non-existent object so that a NeRF can create its 3D version. Companies like 3dfy.ai and Sloyd even use text encoders to allow a 3D modeller to generate these from scratch. The benefits aren’t only on single-asset generating but also on compositing scenes and settings. The next generation of PCG maps are tools like NVIDIA’s GauGAN, which lets you create landscapes from sketches, or LA-based Promethean AI, which allows artists to create virtual 3D environments, simplifying previously quite manual tasks. These files don’t need to slow down the game as well. With K-means clustering for image compression mixed with level-of-detail (LOD) approaches that engines like Unreal provide, all of these object meshes, no matter how detailed the original assets were, can be adapted to reduce the memory usage as the player moves farther away from them. This summer the concept of gaussian splatting was introduced at SIGGRAPH and has been causing a stir, as a means of scene description, so we’re keen on seeing how startups will be using it to create new products in the field.

But this isn’t happening just for visual assets. The world of audio has always been a bit of a pain for game developers, sadly often being seen as a later-stage addition and an expense rather than a point of differentiation. Often games use nightmare-free (aka royalty-free) music for its soundtracks. Perfectly decent music tracks can now be created from a multitude of services, like those of startups like Boomy, Beatoven.ai, Soundful that even the big-guns like OpenAi, Google and Stability.ai have their own solutions. But if the world of music still has some resilience, not being fully substitutional, with commercial music from well-known recording artists and even for original scores commissioned from composers still holding some value, much less can be said about folly, the multitude of audio effects, from car crash sounds to Roblox’s infamous “oof”. Previously the domain of relatively affordable libraries of sample sounds, even the library approach requires audio engineers to search for the right sample, which takes precious time. While there are now tools like IBM’s MAX Audio Sample Generator that allow samples to be promptly created, this is still an area where we see more activity coming. On the other hand, speech, is a field that has had decades of research and work providing a solid foundation for startups like Replica Studios, Reespeecher and Eleven Labs to be able to provide both text-to-speech (TTS) into existing mapped vocal profiles, as well as voice synthesis to create completely new ones. All of this can also be interwoven with the gameplay to create more than just the rigid, trigger-based playing of ‘track 7’ when combat starts and ‘track 8’ when it ends. Startups like UK-based Lifescore and Sweden’s Reactional are infusing a degree of interactivity to sound design, allowing for it to be adapted dynamically to what’s happening in the game.

Source: Reactional
There’s an incredible story of how the phonograph, the record player’s grandfather, was originally marketed across the US, with these showcases where attendees would find themselves in a theatre listening to a superbly-talented opera singer until the lights went off. Bravely, the singer would keep the show going on, but upon the turning of the lights back on, crowds would roar to find that the sound had been coming from a little device that had been laying in a stool next to the singer. It’s almost apocryphal to think the human ear could ever fall for something like that, but our contemporary ear is very different to what our future ear will be. Just in the same way our eyes will be. Crowds getting scared at trains on screen is the proxy to why art directors, 3D modellers, etc. are still concerned with using these tools too liberally in the design process: because future consumers will be more discerning in taste and visual susceptibility than we are today, and they might just spot what’s ‘AI’ generated with a less than merciful look.
Animation

Source: MetaHuman Animator
Things start to get bogged down when you need to make them move. Static object creation is a much easier challenge in comparison to all of the component pieces of animation. Traditionally this has been a process that involved keyframe animations, for both body as well as face, which was a painstaking process that could take weeks depending on the complexity of the animation. This is why motion capture methods emerged as alternatives, using reflective suits and optical cameras, to allow for performers to act the needed movements instead of manually modelling them. But, both because of the hardware as well as the studio space required, this is often an expensive endeavour, so with the advances in pose estimation and image recognition, startups like Move.ai (an FOV Ventures investment) are now able to use deep-learning to identify body parts as they move in video and transform it into an animation file that can be loaded onto a game engine. Unreal itself, as part of its MetaHuman tool has released MetaHuman animator, which uses markerless facial capture to recreate the expressions of an actor in front of a camera. In case you don’t have access to or budget for performers, the next step in this production evolution is what startups like Speech Graphics are doing. They use a labelled facial animation dataset to associate the facial movements that are made when a human emits certain sounds and then, infers from an audio file of a person speaking the needed facial expressions to be able to express that sound. And there are already startups using databases of labelled kinetic as the training set for a generative solution that, upon a descriptive prompt, can do text-to-animation. While the methods of Move.ai and Speech Graphics might not, at all times, deliver a totally clean and fluid animation, they are significantly price-competitive to traditional mocap or facial capture solutions and they massively free up animators’ time to be spent on other parts of the production pipeline. Despite the promise that these solutions hold, there are still concerns from the user base. At the end of the day, it’s a compromise between quality and speed at which point comes scale: if you’re applying hundreds of assets through an animation pipeline, the benefits will definitely outweigh the costs; but if you’re focusing on one primary asset, for example, the perceived gains in efficiency are often misleadingly outweighed by the switching costs of learning, testing, and introducing a new technology into an established workflow.
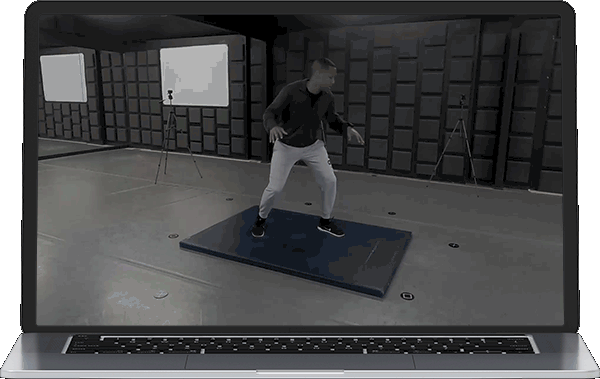
Source: Move.ai
And then there’s the scope problem. While these technologies certainly help make the animation pipeline more efficient, they represent but a fraction of that pipeline, potentially creating issues at other stages in it. Rigging and retargeting animation files onto skeletons fall into that bucket. We’ve certainly seen how developers are starting to be able to create 3D models and animation files, often from nothing, but that doesn’t mean that those two automatically, as if magically, fit each other. This is where retargeting the animation to a rig is still quite a manual problem. Add to it, that if you’re creating a 3D model from nothing, it’s not like it’s going to be created with any rig information, which is why many 3D modellers still prefer to start from a model that has rig data associated with it, shunning some of the generative approaches. This is what products like AccuRig and Anything World’s ‘Animate Anything’ are trying to solve for: the ability to import a non-contextual 3D asset through a code-base that is able to identify not only limbs but bones, joints and how they would work together. But, still, they are but a small portion of the game designer’s job, who ultimately needs to bring both assets and animations into coherent game mechanics, with the help of the game developers. Some might say that is the core of what a game designer is meant to do in their job, so is this really a place that ‘AI’ should enhance? Naturally there are already folks coming out to the market with the firm belief that yes, designing and implementing game mechanics is an area that can be aided by generative neural networks. It’s still unclear as to what level of efficiency, but startups like Unakin are allowing anyone to transform text prompts into game code base, enshrining basic template game mechanics through simple triggers. Things like make Enemy 1 run around in area X, and trigger Explosion Animation 1 and reduce HP by 50 if Player goes within 2 metres of Enemy 1.

Source: Voicebot
The ambition is clear, lowering the barriers to creation will make more talent come into the field and will increase the diversity and possibly the quality of the creative. If that ambition isn’t fulfilled, the same approach can be used by game developers for the purpose of playtesting how simple game mechanics work before launching into full production. But Roblox has proven that there are legs to the ambition of bringing in game development to the masses. And with the most recent news coming out of the Roblox Developers Conference in San Francisco, we see that they are intent on further lowering the barriers, by launching a chat function that allows game developers to almost sidestep Lua - its programming language - in favour of the chat to create environments and behaviours.
3. Player Assistance
Finally we arrive at an area that has been in controversial development for quite a long time, but is now really getting a boost. As mentioned previously, in the early days of gaming, player assistance was synonymous with the walkthroughs published in specialty magazines. During the days of the much-loved, text-based multi-user dungeons (MUDs), scripts started emerging that chained commands together based on text-triggers, easing the burden of a player having to type every single command. As gaming evolved, the player assistance sphere timidly moved to the domain of mostly browser-centric, turn-based strategy games, such as the likes of “Lords” or “Europe1300”. These games, no more than glorified social-probability spreadsheets, were ripe for out-of-game calculators masked as ‘companion apps’, which ultimately catered for the min-max archetype of players out there, who wanted to make sure to be making the best decision possible, with the information available to player as well as estimates based on collective data gathering. All of these were meant to sharpen the edge that a player had against the challenges the game environment threw at them or against other players. And herein lies most of the controversy. While the pieces described so far have never been frowned upon by game developers, as soon as you get into the world of macros and mods, that’s certainly not been the case.
Policing
Macros can be broadly described as software that uses game events to somewhat automate tasks, usually repetitive ones, for the player to concentrate elsewhere. “Runescape”, the popular game from Jagex, has been rife with macros’ use across its player-base, notwithstanding because it’s originally a java browser-based game and so game events can be easily accessed via the code-base, but because it is a clicker, or more depreciatively, a grinder, therefore their use is particularly helpful. Mods, on the other hand, have both fallen by the cosmetic wayside - who doesn’t remember playing “Counter Strike” in “Lord of The Ring”’s Helms Deep map? - as well as those which alter the variables of a game, like ‘god modes’ that can make you fly by altering the physics of a game. Traditionally a lot of game developers, particularly those in the FPS genre, have mostly understood the existence of mods as a nuisance - one which they knew the player-base used but definitively banned for any online multiplayer mode. The reason being that anything other than the vanilla version of a game would not be able to keep a level playing field for all players. One of the advantages that came from the shift from open-access games to launcher-based games is precisely that harmonisation, with companies like Overwolf and products like Steam’s Workshop working to provide compatible mods. Despite that, cheating software has been able to find cracks to give players an upper hand. And this where ‘AI’ is breaking ground. On both sides of the fence. It was the advance in supervised learning approaches for object recognition that allowed the rise of aimbots and triggerbots that identify enemies in the field of view of the player and fire a directional shot. But it’s because of these, that anti-cheating software has also been using the same technologies. Products like Anybrain use deep learning for anomaly detection, in assessing things like the probability player aim, particularly around walls and objects, or even what the player’s normal behaviour tends to be (as it is unconsciously changed when cheating software is used). But, as with anything that has two sides with access to the same technologies, will there ever be a fool-proof solution to cheating?

Source: Crooked Arms
But policing isn’t just about cheating. Indeed, simultaneously one of the most wonderful and saddest parts about gaming are the people. Multiple debates and controversies, from ‘Gamergate’ to “The Last Of Us II”, have undoubtedly proven us that the toxicity of some gaming communities is unrivalled. And rampant. This isn’t just a moral problem. It’s an economics one. Despite the good will of some publishers, this behaviour typically comes from a hardened, hard-core player base, which might typically be big public proponents and big spenders. But the abuse that new players receive increases churn and n-day retention massively. And the harassment that those who express a differing point of view can be subject to, significantly hamper logins, play hours and session times which are intrinsically linked to expenditure. This is why companies like ggwp and Modulate are stepping in, to help police anything from online out-of-game communities to in-game behaviours. We’re keen to see not only those acting with a reactionary approach to incidents, but how deep learning can be use to identify behaviour patterns in order to prevent abuse before it is attempted to be inflicted.
Coaching
With the development of teams and leagues into the increasingly competitive eSports ecosystem though, particularly those with extremely attractive rewards, it’s but an obvious conclusion that a litany of software - that would fall outside of the cheating category - would rise to take hold of all of that latent demand. Following in the footsteps of the old companion apps, like Spirit AI’s Ally, Migame.gg or even games’ own products like Call of Duty’s second screen app, there’s been a significant trend in this space. Startups and products like Omnic.AI, SenpAI, Osirion, and many more ultimately have relied on deep learning for in-game analytics, which can be interpreted via game events. Ultimately, this is software that is meant to be used outside of competitions, in training so as to improve executions of strategies, perfecting methings, as opposed to dynamically alter tactics upon facing certain strategies from opponents in competition. The evolution of this software relies on computer vision techniques that extract information from the screen alongside the game events, as a third eye for the players. This is possible because of dimensionality reduction, which in unsupervised learning approaches, allows the machine to reduce the number of data inputs to a manageable size while preserving data utility for the purpose of analysis. We’re excited to see how much information can be processed in real-time and spit back to the player as helpful tip, although that promise is currently quite hard to execute due to the laggy times that non-locally-stored algorithms have. But, the hope is that the addition of a dynamic, real-time machine, like a rally co-pilot, coaching you through all data aspects of the game will be not too far away. Just not here yet.
In Conclusion
Using AI within gaming is nothing new, but recent advancements have opened up new possibilities. But will outcomes live up to the hype?
Established players like Roblox and Unity all have their attention on the space, whilst funds like FOV Ventures scout through all the new startups that have appeared recently. Who will properly deliver on their promise is still an unknown. But we’ll be watching the space with interest.
Here’s a quick overview of just some of the companies. And if you’re tackling a big problem in this space then we’d love to hear from you.

AI in Gaming Market Map